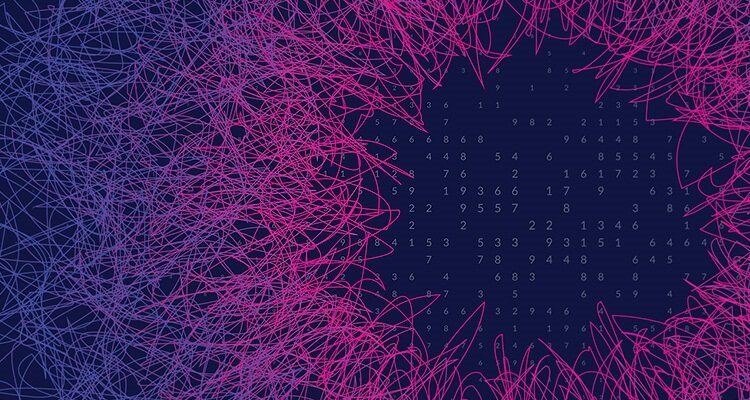
Integrating AI can be costly and time intensive. These four guidelines will maximize its returns
For Anna-Katrina Shedletsky, AI is old tech. An ex-Apple engineer who saw firsthand the inefficiencies of building high-quality products, she recognizes that while the hype for AI may be new, the technologies it’s built on — computer vision, advanced statistics, and machine learning — have been in use for decades. But as she highlights, recent innovations in Systemic AI can provide cutting-edge insights and big results.
The state of AI in manufacturing
AI, the ‘new old thing’ in manufacturing, has applications in new product development, design, operations, field quality, and more. It generally falls into two* main categories:
- Component AI: AI that’s been targeted to solve a specific problem for a specific process in the manufacture of a product – for example, using computer vision to do a visual inspection
- Systemic AI: AI that provides value across a system, like optimizing a production line, typically using multi-variant or multi-modal inputs
*A third kind of AI, General AI, promises to plug into a data lake of messy and unstructured data and magically find insights. This is, at least for now, still science fiction.
Component AI has been around a long time, but Systemic AI is what’s next. “At Instrumental, our Systemic AI focuses on product quality and efficiency. We’ve seen customers leverage AI as their global quality system of record, proactively discovering new issues, accelerating operator training, and preventing escapes. They have saved millions of dollars with double-digit return on their investment,” Anna says. Efficiency is something every manufacturing organization can get on board with – but how to get started?
In Anna’s experience, companies who take the following four steps in roughly this order best set themselves up for success:
1 Build a business case for integrating AI
To justify the time and expense of implementing AI, you should build a case for how it will ultimately increase profits or reduce expenses. You should identify at least three value drivers, such as decreasing headcount, increasing yield, and reducing scrap. Then, build a calculation of the expected savings starting from the metrics you’ll be able to move with the technology. This forms the basis of a return on investment (ROI) business case to justify the expense of implementing a particular technology.
2 Get the data (in the cloud)
It’s not uncommon for useful manufacturing datasets to be siloed, but AI has to be trained on a dataset — images, videos, functional test results, or any metric important to your value drivers. Data, therefore, needs to be centralized in a structured way that’s easily accessible. You might believe you should do this step yourself first, but Anna advises working with a partner who will not only aggregate this data with a drop-in infrastructure but will be responsible for getting value out of it. An internal DIY effort rarely bears fruit because that second part is missing. Aggregating and organizing your data is crucial for accessing AI’s most powerful insights.
However you aggregate that data, Anna argues, it should be in the cloud. This enables data aggregation across many physical locations, remote administration and oversight, remote access for the engineers using the data, and the highest likelihood it will be futureproofed to leverage further advancements. Even regulated manufacturers are leveraging cloud data for these reasons.
3 Select AI technologies that easily provide value
Leverage your business case to select AI technology that will clearly move the needle on your value drivers. Some technologies require you to have your own AI engineers or data scientists to really make use of them. Unless you already have these people on your payroll, don’t buy a tool that requires them. Choose an AI use case that will be easy to train and can leverage your team’s existing expertise.
4 Establish Proof of Value
Design your implementation of the AI technology as a Proof of Value (POV), not a Proof of Concept. A Proof of Concept shows that the technology can do what it’s supposed to (like collecting the metrics it was instructed to collect), but Proof of Value focuses on how this technology influences the value drivers in your business case. If your chosen technology isn’t increasing yields or reducing scrap as expected (and at this point, you’ll have the concrete data you need), you’ll know before you make a large investment.
Realizing value with Systemic AI
Systemic AI technologies for manufacturing can be incredibly powerful and relatively quick to enable if you choose the right partners and take a value-first approach. “This is a really exciting time to be in manufacturing – when set up well, AI can be superhuman and really reduce the cost of quality.”
For a list of the sources used in this article, please contact the editor.
Anna-Katrina Shedletsky
www.instrumental.com
Anna-Katrina Shedletsky is an ex-Apple engineer who saw firsthand the inefficiencies of building high-quality products. She founded Instrumental to build technology to solve that problem with data and AI. Instrumental is now a leading manufacturing quality software provider for hardware brands and manufacturers, optimizing their assembly, operations, and quality control processes. The world’s most admired brands, including Axon, Bose, ChargePoint, Cisco Meraki, and SolarEdge, rely on Instrumental’s cloud platform and purpose-built AI to accelerate time-to-market, improve yields, eliminate rework, and save engineering time.