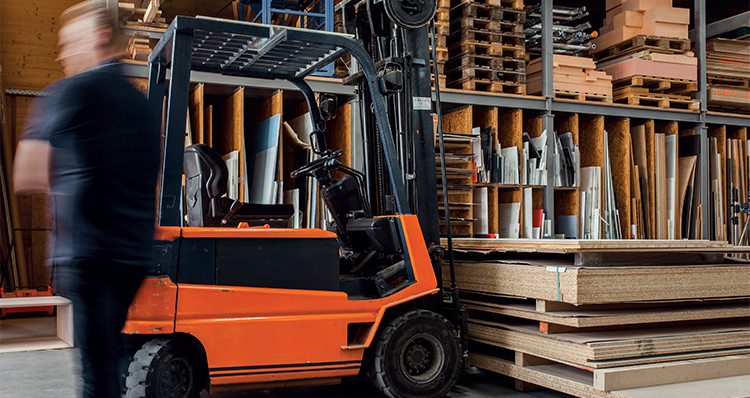
Using data to drive resiliency
Prior to the pandemic, global supply chains relied on tried-and-tested models of manufacturing and forecasting. The Just- In-Time (JIT) model was immensely effective at managing and reducing inventory on hand, while forecasters used time series techniques and years of sales data to produce reliable demand projections to assist with planning over the medium and long term.
But Covid-19 and a series of subsequent disruptions have uncovered the core flaw behind Just-In-Time models – they simply cannot adapt quick enough to variations in supply and demand outside of highly specific parameters. Major disruptive events continue to happen with increasing frequency and, as a result, supply chain managers must consider new techniques and focus on developing a resilient operating model if they hope to mitigate future disruption and swings in demand.
The pandemic – and associated occurrences – have caused the bullwhip effect to grow significantly in recent months. The impact from demand distortions and shifting lead times have travelled upstream through the supply chain – much like a whipcrack – to impact manufacturers directly. These challenges have continued through 2021, even as the successful rollout of Covid-19 vaccinations and the lifting of national restrictions has seen economic growth recover.
The UK is, for instance, currently dealing with a shortage of an (estimated) 100,000 HGV drivers that has been linked to empty supermarket shelves. Meanwhile, the well-documented computer chip shortage has impacted the production of a vast range of goods from cars to consumer electronics. There is now huge competition to not only acquire the raw materials needed to make products, but also issues around how to actually distribute stock once it is made.
Using data for insights: strategies for risk mitigation
So, what needs to change? Building resilience into supply chains is essential but will also be a challenge after years of optimization that has peeled back disruption-absorbing buffers and flexibility to reduce costs. A better way of tracking shifts in supply and demand is also needed, as well as planning more effective responses to disruption. While it is difficult to predict a pandemic or labor shor tage, the failure to properly prepare and plan for such events is an indicator of a non- resilient supply chain – one that is no longer fit for purpose in a world of constant disruption.
Organizations do not have the luxury of waiting for problems to occur before acting; they must plan ahead for the risk of severe disruption to operations. In doing so, the use of existing data and the discovery of additional information to build transparency across their supply chain is paramount. This will help them to develop an understanding of their exposure, vulnerabilities, and potential losses, which will inform their resilience strategies and provide them with the building blocks for crucial insights.
Quality data is integral to this process of mitigating risk. Businesses looking to grow and recover in the post-pandemic economy must consider how they can leverage data analytics to produce powerful insights in real time which will help with improving day-to-day operations, planning for known disruptions and reacting to emergencies. Data is also key to predicting how customer demand will shift during disruption.
By leveraging external data sources – such as global weather forecasts, traffic information, public or cultural holidays, and any potential strike action – as well as internal data, companies can better understand their supply chains and what factors are influencing time to delivery. Applying analytics to this data will also enable companies to observe all aspects of their products, customers, and channels to understand how to better dynamically forecast demand through potential disruption and associated increases in demand following shortages, then adjusting their supply to meet those needs. Doing this will help to create a ‘customer-centric’ supply chain: recent research by Accenture found that ten percent of companies can achieve 13 percent more growth over competitors by adopting customer-centric supply chain practices.
Dynamic demand forecasting enables a business to simulate different scenarios based on a range of variables, which can be compared to reduce risk and provide valuable data-driven insights, such as where the vulnerabilities, bottlenecks and risks exist in a supply chain. By using dynamic demand forecasting to prepare contingency measures for shock disruptions, businesses can start to mitigate the bullwhip effect and build more resilient supply chains.
Leveraging the power of automation to build resilience
For this to work in practice, supply chains need to be agile. Decisions must be made quickly as soon as (if not before) disruption strikes. Dynamic demand forecasting works by using existing data to simulate product trends and purchasing patterns to predict demand. By helping to analyzing all available data and modelling future scenarios, this insight-driven forecasting can be automated to quickly highlight information points which signal disruption, working in tandem with information on alternative suppliers, trade routes, or additional production capacity that can be taken advantage of elsewhere.
To leverage dynamic demand forecasting, businesses must embrace analytics, intake data from external as well as internal sources, and develop transparency across their supply chain. Combined, they will be able to more accurately model demand over the short and immediate term when demand may spike or drop due to disruption.
Businesses also need data-driven analysis to produce insights and identify which goods, routes or suppliers experience the greatest volatility. By anticipating where the cracks in their supply chain might appear, businesses will be able to prepare for when the next major market disruption strikes. Businesses that have modelled for every possible scenario will know how best to respond, providing the agility and resiliency that today’s modern supply chain demands. As global manufacturing sees the early signs of recovery, dynamic demand forecasting offers companies a path to develop resiliency ahead of inevitable future disruption.
Richard Timperlake
Richard Timperlake is SVP, EMEA at Alteryx. Alteryx, the Analytics Automation company, is focused on enabling every person to transform data into a breakthrough. Alteryx unifies analytics, data science and business process automation in one, end-to-end software platform to accelerate digital transformation and shape the future of analytic process automation (APA™).
www.alteryx.com